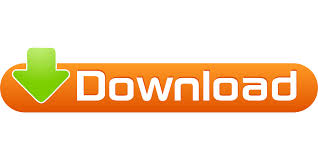
Populism, media, and misinformation in Latin America Ignacio Siles, Larissa Tristan, and Carolina Carazo Latin America has had a long, complex, and complicated relationship with populism.In practice, depending on the multivariates that need to be generated, it may be necessary for these variates to have a degree of dependence (something that I have not discussed in my implementation). In implementing the Latin hypercube method, I have conveniently assumed that the variates generated across each dimension are done independently. For example, since one of the selected pair comes from stratum 1 in both the dimensions, there would not be any further sampled pair that would contain stratum 1 even as an ordinate of the pair. The three sampled pairs can be intuitively represented as shown in Table 4.4.Īs can be seen in Table 4.4, any selected pair provides the only contribution to the stratum inherent in the pair. Furthermore, what is presented in Table 4.3 is just one way to implement this in Microsoft Excel although, in practice, there are other, better ways to do this especially when the size of strata selected ( n) gets large. The implementation of this is shown in Table 4.3.Īs can be seen from Table 4.3, the 3 simulated pairs are (0.74685, 0.34243), (0.04615, 0.04105), and (0.41683, 0.74714) – where the strata are randomly selected in rows 2 to 4 and 9 to 11. To get the actual two-dimensional variates, one randomly generates a number from each randomly sampled stratum – eventually ending with three pairs of two-dimensional, uniform numbers. Repeating this selection process over the remaining strata two more times, one can arrive at another two pairs of the two-dimensional strata. One can randomly pick a stratum in each dimension – ending with a randomly selected stratum pair. As in the stratified sampling method, there would be three equal strata defined by the intervals (0, 1 /3), (1 /3,2/3), and (2/3, 1) in both the dimensions. Given the above backdrop, I will now show an application of this method when generating three two-dimensional uniform numbers. Once this is done, the process is then repeated over all the remaining strata in each of these dimensions, so as to end up with n d-dimensional numbers – which was what McKay, Conover, and Beckman proposed with their latin hypercube sampling method. If only n (and not nd) d-dimensional numbers are required, one can randomly pick a stratum (from the n strata) for each of the d dimensions and then select a random number from each of the selected stratum to end up with a d-dimensional uniform generated number. Clearly as the dimension grows, so does the amount of computational time required to generate such numbers – resulting in an inefficient way of extending the stratified method.
#Latin hypercube sampling vs random sampling pdf#
Thus, if one needs to generate a variate from a multidimensional uniform pdf (with dimension d) one can simply divide the (0,1) interval across each dimension into n equal-sized strata and then generate a number in each stratum – resulting in nd tuplets (where each tuplet is d-dimensional). To generate uniform numbers for higher dimensions, one could simply (and blindly) extend the stratified sampling method to higher dimensions. In fact, Latin hypercube sampling tends to be a more powerful and efficient method than the stratified sampling method when generating multidimensional variables. While this method is identical to the stratified sampling method when generating variables from univariate distributions, the process undertaken in this method to generate a multidimensional variate is slightly different from that using a stratified sampling method. McKay, Conover, and Beckman first advocated this sampling method in 1979 to deal with the generation of variables from multivariate distributions.
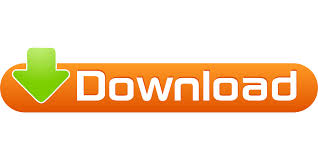